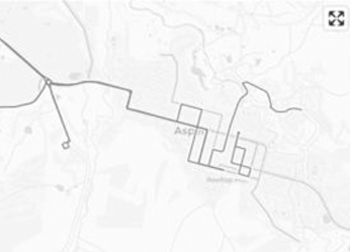
Passive Data Collection Passive Data Collection Vendor Types
- Date: January 5, 2023
Jump to section
As described previously, there are various types of passive data collection technologies, with each providing differing use cases for the transit agencies that utilize them, as well as differing mthods of how these data are collected. These can range from utilizing “big data” vendors (who use the aforementioned “Smartphone” enabled systems to gather data) to vendors of hardware systems that are provided “on-site”.
In October and November of 2021, interviews were held with vendors of passive data gathering systems – StreetLight Data, Transit App, and Avail – to gather information and examples regarding the capabilities of their technologies and how passive data is being gathered and utilized in transit. The companies provide products that serve different business functions, but all three generate and/or utilize passive data to provide solutions to transit agencies and riders.
This section of the Guidebook summarizes the information obtained from the interviews to show the overarching capabilities of some of the current technologies as well as some challenges that have been observed in the transition from active to passive data collection.
“Big Data” Vendors (e.g. Street Light Data)
Some companies mine and amalgamate data sourced through passive mobile phone location data and blended it with spatial or statistical data. This data is then refined and presented in a manner that allows practitioners to understand and utilize the data. This process is referred to as “Passive Data-Derived Analytics.”
Data analytics companies serve a variety of business functions, but three common transportation uses include vehicle tracking, last mile studies, and origin-destination data generation. Vehicle tracking is able to obtain speed, demographic, and location data from vehicles, which can be valuable when developing transit network designs and corridor studies as it provides insights to the mobility needs in an area beyond that of average annual daily traffic (AADT) or other more traditional methods of traffic analysis. For example, San Mateo County Transportation Authority utilized vehicle tracking to determine that low bus ridership might have been a result of transit schedules not matching commuting travel time patterns, as opposed to a lack of intrinsic demand for transit service. After adjusting the schedules to better align with travel time patterns, ridership grew by 30 percent.
Their data can also support last mile studies; for example, data from mobile phones can help planners understand demand at park-and-ride lots both in terms of volume, times of use, and mode of access to and from a park-and-ride lot.
These companies can also provide detailed origin-destination information that includes locations and mode selection. For one company to develop software that can identify the mode in which people are traveling, contractors were hired to record their mode of travel (by keeping a “diary” of their travel); this data was subsequently used to develop a training set, which teaches the machine learning software to correctly classify a person’s mode of travel.
Agencies should ensure that all data used by these vendors is anonymized and, when evaluating bus networks, it doesn’t track individual bus operators; rather, data is provided at the system or route level.
Typical Client
Clients of these companies vary in type and in size; however, traditionally, clients consist of large metropolitan planning organizations (MPOs) or State agencies, and in many cases includes planning efforts for a transit system. However, the number of small and mid-sized organizations utilizing data vendors is becoming increasingly common as big data becomes more engrained in transportation planning processes. This type of data can serve both urban and rural populations – companies have found that their data provides rural practitioners value as passive monitoring is cheaper than in-person data collection methods.
Overall Compatibility and Data Sharing
Look for vendors that have a policy that encourages sharing data. There is flexibility built into the data sharing process, but the general approach for the vendor interviewed is to restrict clients’ ability to aggregate data (whose methods may be viewed as proprietary for a vendor) but to share results. The vendor interviewed, for example, contributes to Open Streets, meaning it shares anonymized data with the public; however, project-specific data can be limited on a case-by-case basis based on the preference of the client.
Benefits and Challenges
This type of business model is built on minimizing agency requirements and does not require client agencies to have a background in data analytics. However, if the user does have data analytics capabilities, the product’s overall utility might be increased. The product is meant to decrease the burden of “big data analysis” on agencies, given it is expensive and time consuming for firms and agencies to collect, store, and process detailed data. If the vendor maintains the data collection software itself, there is no need for localized maintenance efforts by agencies. Some parties also have ethical concerns about government data management practices; in some cases, these can be assuaged when the data is managed and stored by a third party vendor such as StreetLight Data.
As transit agencies tend to gather data from various sources, the lack of standardized data is a significant challenge. Given the lack of standardization, the data vendors’ products work best when paired with OpenStreetMap data. Some vendors are working on developing an industry standard that addresses how to best centralize data.
Finally, the data amalgamation provided by vendors generally focuses on individuals with mobile smartphones, meaning data collected may not fully reflect a given community. The data would be most likely to omit demographic groups that are less likely to have mobile smartphones, such as seniors. Though this means data is not entirely comprehensive for a given study area, most vendors feel the risk of not including at-risk populations and non-smartphone users is limited because traditional methods like in-person surveying also tend to have these biases. Additionally, mobile phone ownership is growing, and the present context is different from previous decades.
Trip Planning/Discovery Application Vendors (Example: Transit App)
The goal of trip planning and discovery applications (or “apps”) are to help people get around their cities, regardless of size, through a display of nearby mobility options in a mobile smartphone application. The app utilizes open source data, including data that agencies publish on vehicle routes, bike shares, scooter locations, and other types of travel modes. The app can also work with data submitted by transit agencies to help their users understand and utilize the various transportation options available. The data is displayed as real-time information to the app user to allow for multi-modal trip planning as well as fare collection.
Apps have deployed directional features as well, such as “GO” in Transit App. These are travel companions in the app that provide the user with audio directions and guidance at different stages of their trip. The app can track when and where the app is opened, when users view nearby routes, trips that users plan within the app, and when and where tickets are purchased. Agencies can also pay to have additional data analytics performed on their data.
Pioneer Valley Transit Authority in Springfield, Massachusetts and the Big Blue Bus in Santa Monica, California are examples of agencies that are incorporating ticketing into these apps to simplify the ticketing and payment system. All data used and collected through the app is anonymized for privacy purposes.
Typical Client
Client agencies are typically smaller urban or rural agencies. Nonetheless, such apps consider agencies with approximately 150 buses their primary client size because they are large enough to benefit from the app and need the assistance.
Overall Compatibility and Data Sharing
Apps only require the presence of cellular data or Wi-Fi for users to access the real-time data and utilize the fare collection component of the app. Some are also compatible with kiosks, which could mitigate accessibility concerns as some demographic groups are less likely to have mobile smartphones for use of the app.
There are agreements that client agencies must sign with a consultant before they share data, as there are rules around data aggregation, anonymity, and privatization which must be complied with. The apps offer ways to provide data to a client regarding route reliability and ridership levels.
Benefits and Challenges
Trip discovery and planning apps combine real-time route information, multimodal trip planning, fare collection, and audio directions into an app that can be directly used by transit riders. The transit agency does not need any specific settings or staffing requirements to utilize the app; however, having an in-house data analyst is helpful. For the apps to function properly, the transit agency must keep schedules, such as General Transit Feed Specification (GTFS) data, and real-time information up-to-date, as this is what is communicated through the app. It was noted in an interview with Transit App that it is best for agencies to rely on firms with digital expertise to build their schedule files rather than attempting to do this task themselves.
On-Site Collection Tools (Example: Avail Technologies)
Certain vendors provide end-to-end packages for agencies that include hardware, software, and various data collection and analysis services to help an agency passively collect data on vehicle health, passenger counting, and fare collection. The vendor interviewed – Avail Technologies – also provides a public-facing app for smartphones. Most contracts with agencies are full-service bids where Avail goes onsite to conduct a needs assessment, study current practices, provide Standard Operating Procedures, and maintain products in use.
Vehicle health monitoring has become an increasingly important part of some services as the expanded deployment of electric vehicles introduces “range anxiety” and other areas of concern associated with the relatively new technology being utilized. Butler Transit Authority is an example of a transit agency that initially needed a public-facing informational app but then expanded into a much larger scale of data gathering and distribution. This was an example of “growing out” the pieces and capabilities of on-site services and seeing the value in automating various processes.
Overall Compatibility and Data Sharing
These systems import an agency’s scheduling data rather than developing an agency’s schedules themselves. There are many sources for scheduling packages that are meeting the needs of agencies, so products are designed to be as compatible with those packages as possible. Some vendors, such as Avail, generate and utilize numerous variations of data including some protected data, such as payroll information, that cannot be shared. However, all data generated with Avail software or hardware belongs to the agencies and can be used at the agencies’ discretion. All data is stored in the Cloud.
Benefits and Challenges
These systems are able to provide stop-level detail, which can provide critical information when making decisions about changing stops or rescaling a system. By knowing who is riding, when they are riding, and which stops they are utilizing, agencies can make informed decisions that provide benefits to the most people.
Products can also provide detailed data on buses that can indicate if drivers are accelerating or braking too quickly and reducing the fuel or energy efficiency of the vehicles.
All data is provided in real time via cellular networks. While cellular networks are the only requirement, Avail stated in an interview that it is important that agencies also keep their General Transit Feed Specification (GTFS) data as up-to-date and accurate as possible. Issues have arisen that are tied to an underlying schedule problem, which can have downstream impacts and lead to confusion with the data. Additionally, once the services are rolled out, there is a maintenance plan to which agencies need to adhere. If the equipment is not routinely calibrated, it will get “out of sync” and provide inaccurate data.
While some small agencies have done very well with the rapid increase in data, on-site vendors’ service model is designed to fill the gaps so agencies can function seamlessly regardless of size and resources available. However, a current challenge with passive data is establishing trust in the data. The data is not perfect, but the systems are automated and run continuously, meaning the same data is being provided consistently, which can reduce the noise that is included in manually collected data. Building acceptance of automation is currently a challenge and will likely be one of the top issues agencies face when incorporating passive data into their systems and decision-making processes. Many small agencies outsource their information technology (IT) efforts, which leads to the critical need to have a “champion,” ideally in operations, that advocates for data collection and the employment of the tools that are available.
Conclusions
The availability of passive data collection in transit will continue to grow as technology and a focus on the ability to utilize “big data” progress. The vendors interviewed fill different market needs, but they all focus on utilizing data collected passively to provide a product that allows agencies to make more data-driven decisions and for passengers to have accurate “real time” information (and thus have better and more reliable access to transit systems of various sizes and modes). A key shift that is occurring with the rise of passive data collection is the speed in which this data is communicated. More data is being communicated in real-time to both agencies (such as Avail providing vehicle health data), and passengers (such as Transit App providing arrival times and bus locations).
As with all data-driven tools, these vendors rely on accurate and standardized data, making it extremely important to keep underlying data inputs – such as a system’s General Transit Feed Specification (GTFS) information – up to date. This sets the stage for potential future initiatives that would further standardize data reporting processes for agencies on a larger scale.
While the availability of data continues to increase and vendors continue to offer innovative passive data collection capabilities and solutions, there is still a need to cultivate trust in the data, particularly among operations staff at transit agencies. Several of the passive data collection technology vendors interviewed mentioned that it is important for agencies to have a “champion” that advocates for the adoption and utilization of the passive data collection technologies that are available and becoming ever more present.
It is vitally important for transit agencies to keep underlying data inputs that various vendors use – such as the GTFS information – up to date